A new AI model named s1, unveiled in a paper released on February 2, is garnering attention for its cost-effective performance that rivals OpenAI’s o1, achieving significant capabilities at a training cost of just $6.
AI model s1 emerges as cost-effective alternative to OpenAI’s o1
The s1 model reaches performance levels close to state-of-the-art, utilizing simpler infrastructure. It enhances large language models (LLMs) during inference by extending “thinking time” through interventions such as replacing terminal tags with prompts like “Wait.”
Trained on a distilled dataset consisting of 1,000 high-quality examples from Qwen2.5, developed by Alibaba Cloud, s1 employed 16 Nvidia H100 GPUs, with a single training run lasting approximately 26 minutes. The total computational cost was about $6, allowing for more frequent experimentation, even for teams with limited resources.
While larger organizations like OpenAI and Anthropic depend on extensive infrastructure, innovations like s1 demonstrate the potential for progress within constrained budgets. However, the introduction of s1 has sparked concerns regarding “distealing,” a practice where models utilize distilled datasets from other AI systems, raising ethical and legal questions that have ignited industry discussions.
In tests measuring math and coding abilities, s1 performs comparably to leading reasoning models, such as OpenAI’s o1 and DeepSeek’s R1. The s1 model, including its data and training code, is accessible on GitHub.
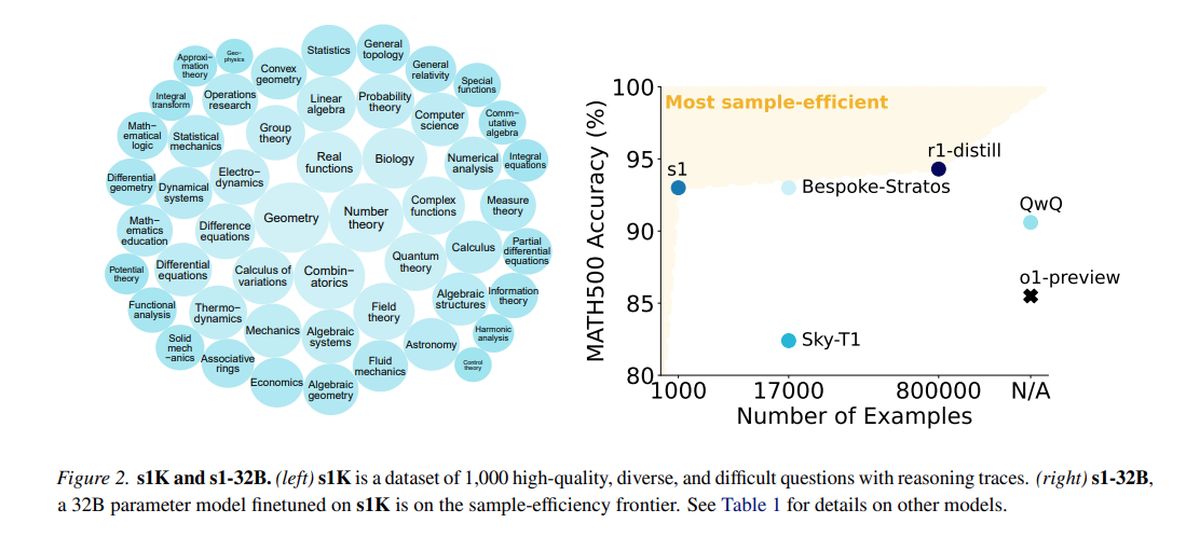
The team behind s1 began with an off-the-shelf base model and refined it through distillation, a method to extract reasoning capabilities from an existing AI model using its answers. Specifically, s1 is distilled from Google’s Gemini 2.0 Flash Thinking Experimental, representing a similar approach used by Berkeley researchers to develop an AI reasoning model for approximately $450 last month.
The ability for smaller research teams to innovate in the AI space without substantial financial backing presents both excitement and challenges. The question arises about the sustainability of proprietary advantages in a landscape where costly models can be replicated affordably.
OpenAI has expressed dissatisfaction, alleging that DeepSeek improperly harvested data from its API for the purpose of model distillation. Researchers aimed to devise a straightforward approach that achieves robust reasoning performance and “test-time scaling,” enabling AI models to engage in deeper analysis before responding.
The s1 research indicates that reasoning models can be distilled using a relatively small dataset through a method called supervised fine-tuning (SFT), which instructs the AI model to imitate certain behaviors within a dataset. This method tends to be more economical than the large-scale reinforcement learning approach utilized by DeepSeek for its R1 model.
Google provides free access to Gemini 2.0 Flash Thinking Experimental, though usage is subject to daily rate limits, and its terms prohibit reverse-engineering models to create competing services. The s1 training process involved curating a dataset of 1,000 tailored questions and answers along with the reasoning processes derived from Gemini 2.0.
Following training, which took less than 30 minutes, s1 demonstrated strong performance on specific AI benchmarks. Niklas Muennighoff, a researcher at Stanford involved in the project, indicated that the necessary computing resources could be rented today for about $20.
The researchers also implemented a technique to enhance s1’s accuracy by instructing it to “wait” during reasoning, thereby extending its thinking time and achieving slightly improved answers.
Featured image credit: Google DeepMind/Unsplash