Mistral AI made Le Chat available to everyone, bringing another AI chatbot into the mix. OpenAI’s ChatGPT, particularly its free version, has long dominated the AI assistant space, but Mistral is betting big on its faster responses, deeper web integration, and more flexible AI capabilities.
Mistral Le Chat vs OpenAI ChatGPT
So, how do these two free AI chatbots compare? We break down the differences in features, performance, and real-world usability to see if Le Chat is finally a worthy ChatGPT alternative—or even a better choice.
DeepSeek R1 vs o3-mini in performance, cost, and usability showdown
Performance and accuracy
When it comes to speed and reasoning, Le Chat and ChatGPT take different approaches. Mistral AI claims that Le Chat is the fastest AI assistant, delivering responses at up to 1000 words per second with its Flash Answers feature. Meanwhile, OpenAI’s GPT-4o mini balances speed with thoughtful, structured responses, though it lacks the same real-time quickness that Le Chat advertises.
Response time
Le Chat’s Flash Answers allow near-instant responses, particularly for straightforward queries. This makes it useful for quick lookups, brainstorming, or rapid summarization tasks. However, this speed may come at the cost of depth, as faster models sometimes provide shorter, less nuanced answers.
ChatGPT’s GPT-4o mini takes a more measured approach. It doesn’t prioritize ultra-fast delivery, but instead aims for coherent and detailed responses, making it more reliable for complex reasoning and structured writing tasks.
Knowledge
Le Chat integrates information from real-time web sources, breaking news, and social media, giving it an advantage in timeliness. For users looking for current events, stock trends, or real-time sports scores, Le Chat may provide more relevant and up-to-date insights.
ChatGPT, on the other hand, relies on pre-trained knowledge and has limited access to web browsing. While it can still fetch recent information, it doesn’t actively prioritize breaking news or integrate as many external sources. However, its knowledge base is often more structured and reliable because it avoids pulling from unverified sources.
Code execution and data analysis
Both assistants offer code execution and advanced data analysis, but Le Chat takes it a step further with in-place code execution, OCR document scanning, and superior file processing capabilities. Its ability to analyze PDFs, spreadsheets, and log files with high accuracy gives it an edge for users who frequently work with documents and technical data.
ChatGPT, while capable of code execution and data analysis, offers limited access to these features in its free tier, meaning users may need ChatGPT Plus to unlock full capabilities.
User experience and ease of use
When it comes to UI design, navigation, and usability, both Le Chat and ChatGPT Free take a minimalist approach, but with different priorities.
Interface and navigation
- Le Chat: The interface is simple and centered around functionality. It prominently displays core features like Canvas, Web Search, Image Generation, and Code Interpreter in the main input box, making it clear what’s available. The left sidebar provides a history of past interactions, similar to ChatGPT. The pixelated “M” logo at the center gives a unique brand identity but doesn’t add much functionality.
- ChatGPT: The UI is sleek and clean, with an inviting “What can I help with?” prompt. Unlike Le Chat, it suggests task categories upfront, such as Analyze Data, Summarize Text, Brainstorm, and Make a Plan, making it more user-friendly for beginners. The voice button is also easily accessible, which Le Chat lacks.
- Le Chat puts core AI features inside the input box for easy access, while ChatGPT separates them into icons above the chat.
- ChatGPT’s UI feels more polished, with intuitive categorization of tasks, while Le Chat prioritizes direct access to its advanced tools.
- Both provide a left-side panel for chat history, but ChatGPT’s design feels less cluttered.
Data connectors and customization
One major upcoming difference between the two services is their approach to automation and customization:
- Le Chat is planning to introduce data connectors and multi-step automation, allowing users to integrate it into their workflow (e.g., connecting to emails, databases, and messaging systems). This could make it a stronger productivity tool once released.
- ChatGPT Free already supports Custom GPTs, allowing users to personalize AI behavior for specific use cases. While this is more suited for developer and advanced users, it provides immediate customization benefits.
Task 1: News research
Le Chat provided a well-structured financial breakdown of Bitcoin’s latest market movements, dividing the summary into sections like Market Volatility, Trade Tariffs Impact, and Investor Sentiment. The response emphasized specific price changes and macroeconomic factors, such as Donald Trump’s trade policies, which were linked to cryptocurrency declines. However, while the summary was organized, it lacked deep source integration—only citing AFP News and FXStreet without direct article links. This makes Le Chat effective for users who need quick, structured insights, but less useful for those seeking broader analysis or verifying information through multiple sources.
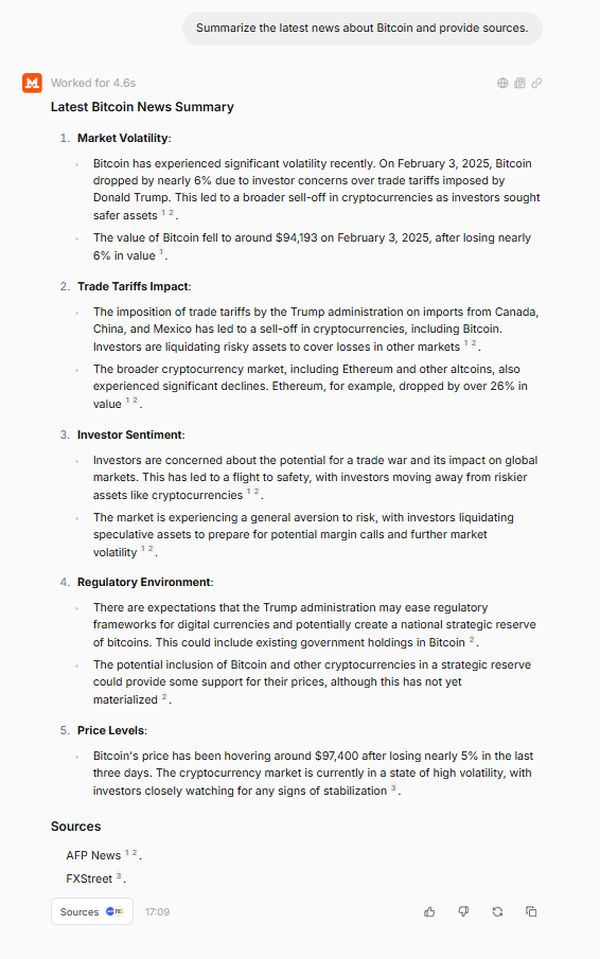
ChatGPT, in contrast, delivered a more contextual and narrative-driven summary, focusing on institutional investments, ETF inflows, and broader market sentiment rather than just percentage drops. It referenced MicroStrategy’s growing Bitcoin holdings and delayed U.S. tariffs, adding long-term financial trends to its response. A major advantage was source diversity—ChatGPT cited Yahoo Finance, The Economic Times, and Investor’s Business Daily, linking directly to recent articles. This makes it better for users who want a comprehensive macroeconomic view rather than a strict financial snapshot.
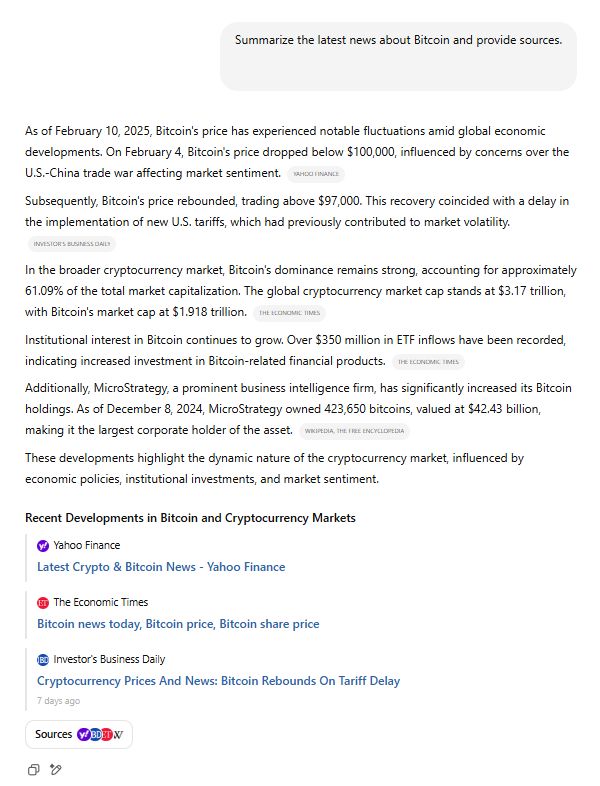
Task 2: Image generation
Le Chat delivered a stunning, highly detailed, and artistically rich image, making full use of Black Forest Labs’ Flux Ultra model. The lighting in the scene is natural and cinematic, with soft shadows and warm highlights that create a sense of realism. The textures are crisp, from the boy’s clothing and hair to the fine details in the dog’s fur and the forest environment. The composition is well-balanced, with depth of field and perspective handled expertly—the focus remains on the subjects while the background subtly fades. This level of quality is closer to high-end digital artwork than a typical AI-generated image, demonstrating Mistral’s strength in image synthesis.
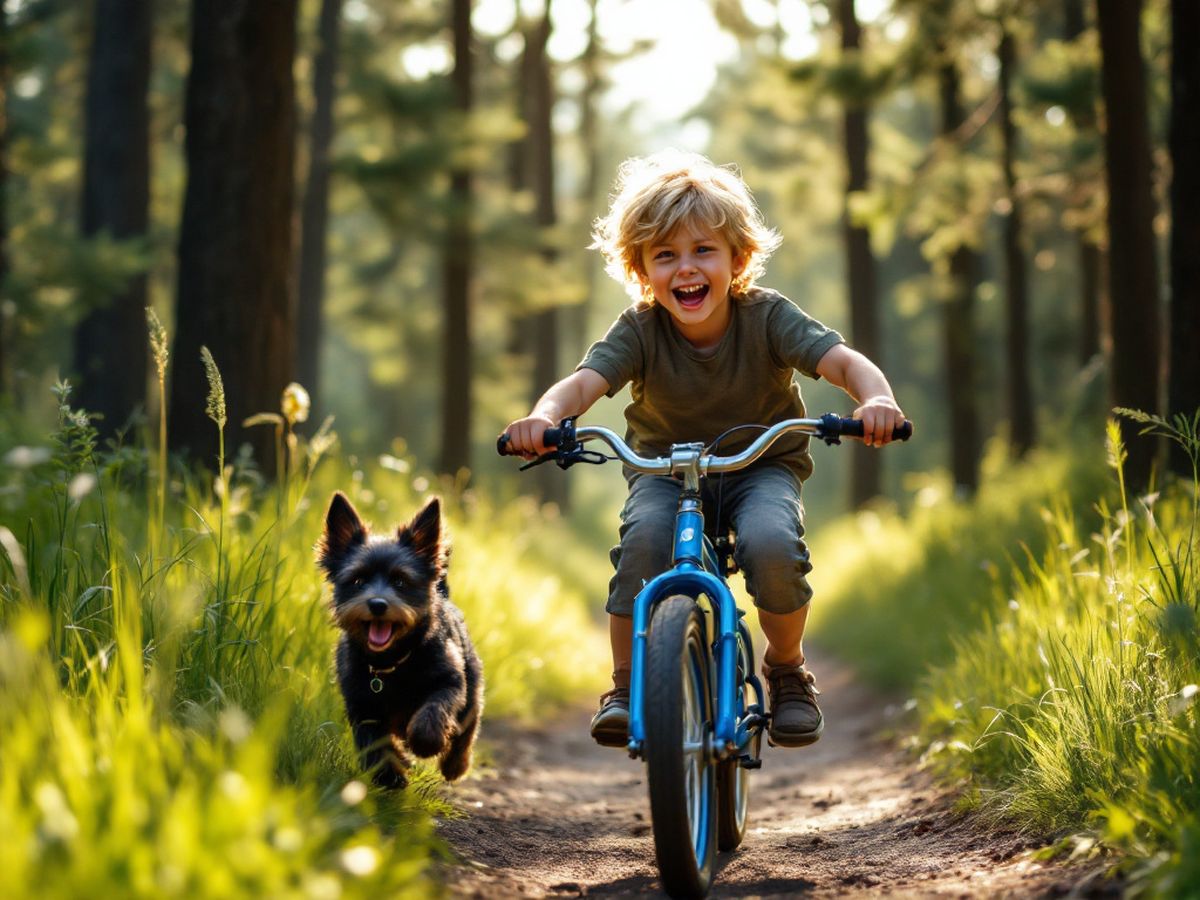
ChatGPT’s image, on the other hand, falls far behind in quality compared to Le Chat. While it captures the basic elements of the scene—a boy riding a bike with a dog—the execution feels far less refined. The lighting is flatter, the depth and sharpness are noticeably weaker, and details such as facial expressions and fur textures lack precision. The composition is still functional, but the image lacks artistic richness and photorealistic elements that Le Chat managed to execute flawlessly. OpenAI’s image generation, at least in its free tier, is severely underwhelming, making Le Chat the clear winner for users looking for high-quality visuals.
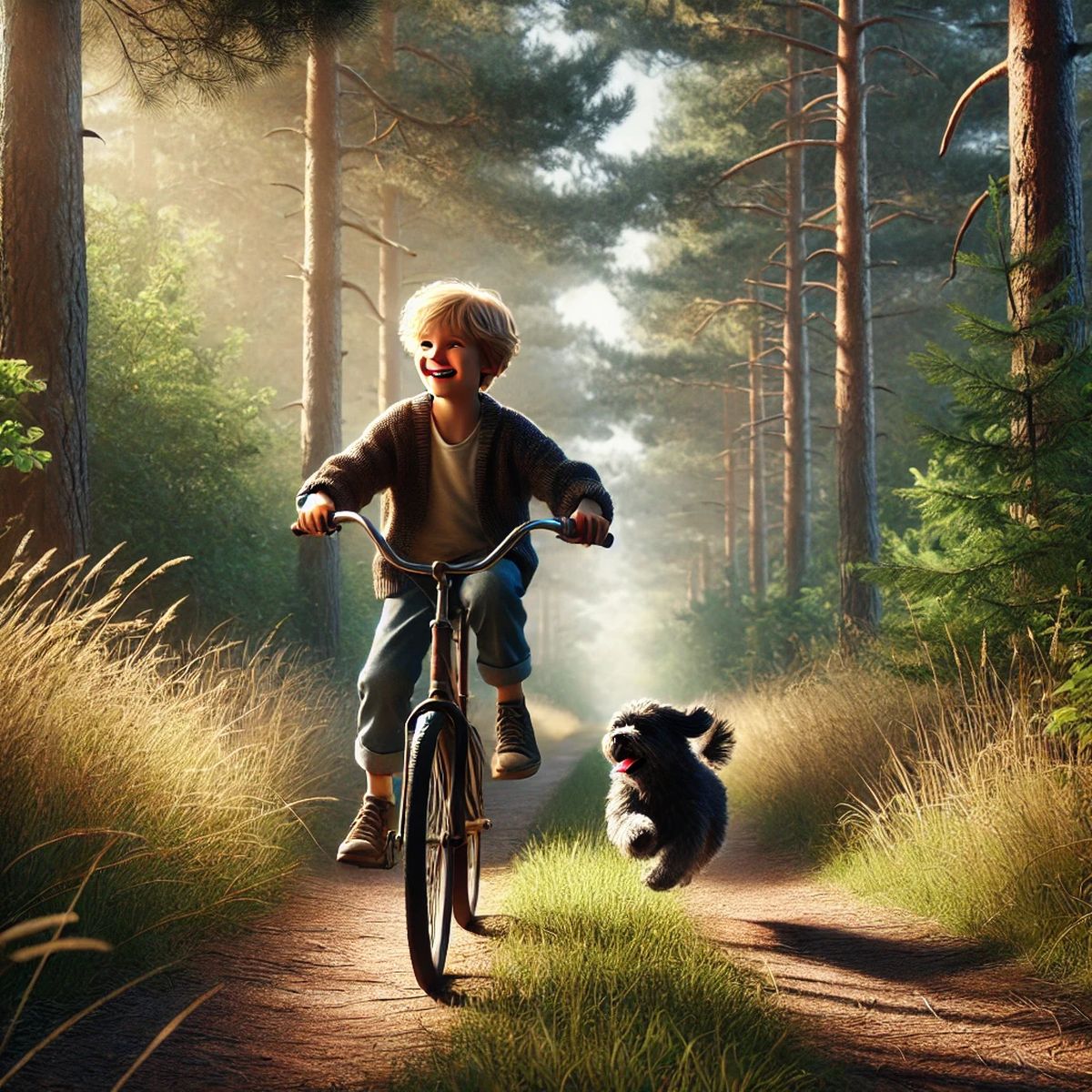
Task 3: PDF processing and summarization
We uploaded this research we covered on Dataconomy and asked for a summarization:
ChatGPT provided a comprehensive and well-structured summary of the research paper, breaking it down into sections like key problem, hypothesis, methodology, findings, and implications. The response was thorough and precise, maintaining all critical elements of the study while ensuring readability. The structure made it easy to follow, and the step-by-step breakdown of Human-Human, Human-AI, and AI-AI experiments highlighted how the paper supported its claims. Additionally, ChatGPT’s version included detailed conclusions and implications for AI safety, making it highly valuable for those seeking a deeper understanding of the research. However, it leaned towards a longer, academic style summary, which may be excessive for users looking for a quick, digestible recap.
Below is ChatGPT’s answer:
Summary of “Scalable Oversight for Superhuman AI via Recursive Self-Critiquing”
This paper investigates scalable oversight mechanisms for AI systems that surpass human cognitive capabilities, focusing on Recursive Self-Critiquing (RSC) as an alternative to traditional human-in-the-loop alignment methods like Supervised Fine-Tuning (SFT) and Reinforcement Learning from Human Feedback (RLHF).
Key Problem & Motivation
As AI models become more capable, human oversight in evaluating AI-generated outputs becomes infeasible for complex tasks, such as long-form text evaluation or high-level mathematical reasoning. Existing RLHF and SFT techniques rely heavily on human supervision, but this fails when AI-generated outputs exceed human understanding.
Hypothesis:
The paper proposes that:
- Critiquing a critique is easier than critiquing an original response.
- Similar to how verification is easier than generation.
- This difficulty relationship holds recursively.
- Higher-order critiques (e.g., critique of critique of critique) offer a structured way to supervise AI at increasing complexity levels.
Methodology & Experiments
The study tests its hypothesis through three types of experiments:
- Human-Human Critique Experiments
- Objective: Assess whether higher-order critique leads to more effective evaluations.
- Findings:
- Higher-order critiques (C2, C3) improve evaluation accuracy while reducing cognitive load.
- Human annotators performed better at evaluating critiques than direct responses.
- Human-AI Oversight Experiments
- Objective: Test if humans can effectively oversee AI using recursive self-critiquing.
- Findings:
- Even when AI-generated responses were beyond human expertise, humans could still effectively critique AI’s critiques.
- Recursive critique enabled humans to supervise AI models that outperformed them in direct problem-solving.
- AI-AI Recursive Self-Critiquing
- Objective: Assess whether AI can autonomously evaluate its own critiques.
- Findings:
- AI struggled with effective self-critique beyond a certain recursion depth.
- Higher-order critiques did not consistently improve AI’s performance.
Key Contributions & Findings
- Verification-based oversight is scalable.
- Instead of directly evaluating complex AI-generated responses, human supervisors can oversee AI-generated critiques.
- Recursive self-critiquing offers a tractable evaluation path.
- Higher-order critiques simplify the supervision of superhuman AI systems.
- Humans can oversee AI through structured critique, even when direct evaluation is infeasible.
- AI struggles with autonomous self-critiquing, highlighting limitations in current recursive critique capabilities.
Implications for AI Safety & Alignment
- AI alignment must move beyond direct human feedback as models surpass human cognitive thresholds.
- Recursive self-critiquing provides a scalable alternative by structuring evaluation into higher-order critique chains.
- Future AI systems may need explicit training in self-critique to improve oversight reliability.
Conclusion
This study provides strong evidence that recursive self-critiquing can enhance AI oversight, reducing reliance on direct human evaluation while ensuring reliable AI alignment. However, current AI models struggle with self-critique, indicating the need for further research into training models for recursive reasoning.
Le Chat’s summary was also well-organized and clearly formatted, but it leaned toward a more concise, high-level explanation of the study. It followed a logical structure, beginning with an overview of the problem, then outlining key concepts, methodology, and conclusions. While it covered all the major takeaways, it did not delve as deeply into the individual experiment findings as ChatGPT did. Instead, Le Chat focused on impact and real-world implications, which might be preferable for readers who want a straight-to-the-point summary rather than a fully detailed analysis. Overall, both AI assistants performed well, but ChatGPT excelled in depth, while Le Chat provided a more streamlined, executive-style summary.
Below is Le Chat’s answer:
Summary of “Scalable Oversight for Superhuman AI via Recursive Self-Critiquing”
Abstract and Introduction
The paper addresses the challenge of ensuring reliable oversight for AI systems that surpass human capabilities. Traditional alignment techniques like Supervised Fine-Tuning (SFT) and Reinforcement Learning from Human Feedback (RLHF) rely on direct human assessment, which becomes infeasible as AI outputs exceed human cognitive thresholds. The authors propose that “critique of critique” can be easier than direct critique, and this difficulty relationship holds recursively. They conduct Human-Human, Human-AI, and AI-AI experiments to validate these hypotheses, suggesting that recursive self-critiquing is a promising approach for scalable oversight.
Key Concepts
- Recursive Self-Critiquing:
- The idea that evaluating critiques of critiques (higher-order critiques) can be more manageable than direct evaluation, especially as AI capabilities advance.
- This approach is inspired by the principle that verification is easier than generation.
- Experimental Setup:
- Human-Human Experiments: Humans evaluate human outputs, examining the progression from response to higher-order critiques.
- Human-AI Experiments: Humans evaluate AI-generated outputs and self-critiques, focusing on tasks where AI outperforms humans.
- AI-AI Experiments: AI systems evaluate their own outputs through self-recursive critiques.
- Findings:
- Recursive self-critiquing shows promise in enabling effective human oversight of advanced AI systems.
- Humans can provide more effective evaluations through higher-order critiques, even when AI surpasses human performance.
- Current AI models struggle with higher-order critique tasks, indicating a need for further development in this area.
Experimental Results
- Human-Human Experiments:
- Higher-order critiques (e.g., critique of critique) consistently improved evaluation accuracy and confidence while maintaining or reducing completion time.
- Recursive critique remained effective up to the third order (critique of critique of critique).
- Human-AI Experiments:
- Humans achieved higher accuracy in evaluating AI outputs through recursive critique, even when AI outperformed them in direct tasks.
- The approach improved evaluation efficiency and confidence.
- AI-AI Experiments:
- AI models showed limited success in performing recursive critiques, highlighting the need for further advancements in AI critique capabilities.
Conclusion
The paper concludes that recursive self-critiquing is a promising direction for scalable oversight of superhuman AI. While current AI models face challenges in higher-order critique tasks, the framework offers a pathway for maintaining effective oversight as AI capabilities continue to advance.
Impact Statement
The recursive self-critiquing framework aims to address scalable AI oversight challenges, promoting responsible AI development by involving diverse stakeholders in supervision. This research contributes to social welfare by advancing scalable oversight mechanisms.
Choosing the right AI for your needs
The Le Chat vs. ChatGPT Free comparison reveals that both AI assistants excel in different areas, making the choice highly dependent on what users prioritize. If speed, real-time information, and powerful image generation are your primary needs, Le Chat is the clear winner. It dominates in news retrieval, document analysis, and artistic visuals, offering faster responses and cutting-edge media synthesis. However, it currently lacks some advanced customization features, such as Custom GPTs, and its citation depth in research tasks is somewhat limited. Users who need quick, structured insights rather than in-depth reasoning will find Le Chat’s efficiency unmatched.
On the other hand, ChatGPT Free provides a more balanced, structured, and reliable experience, making it better for those who value detailed responses, well-organized summaries, and a polished user experience. It may not be as fast as Le Chat, but it offers stronger citations, better contextual reasoning, and the ability to create Custom GPTs, which adds significant flexibility. However, its image generation is subpar, and some features, like advanced code execution and file handling, are limited in the free tier. If you need depth, coherence, and customization, ChatGPT Free remains a strong contender. Ultimately, Le Chat is the AI for fast, media-rich, and real-time tasks, while ChatGPT excels in structured knowledge, citations, and thoughtful AI reasoning.